Research
Foundational Research
ARNI’s work centers on five major conceptual research thrusts that address foundational aspects of strong generalization. These institute-wide areas integrate knowledge, data, and researchers from the fields of neuroscience, cognitive science, and AI.
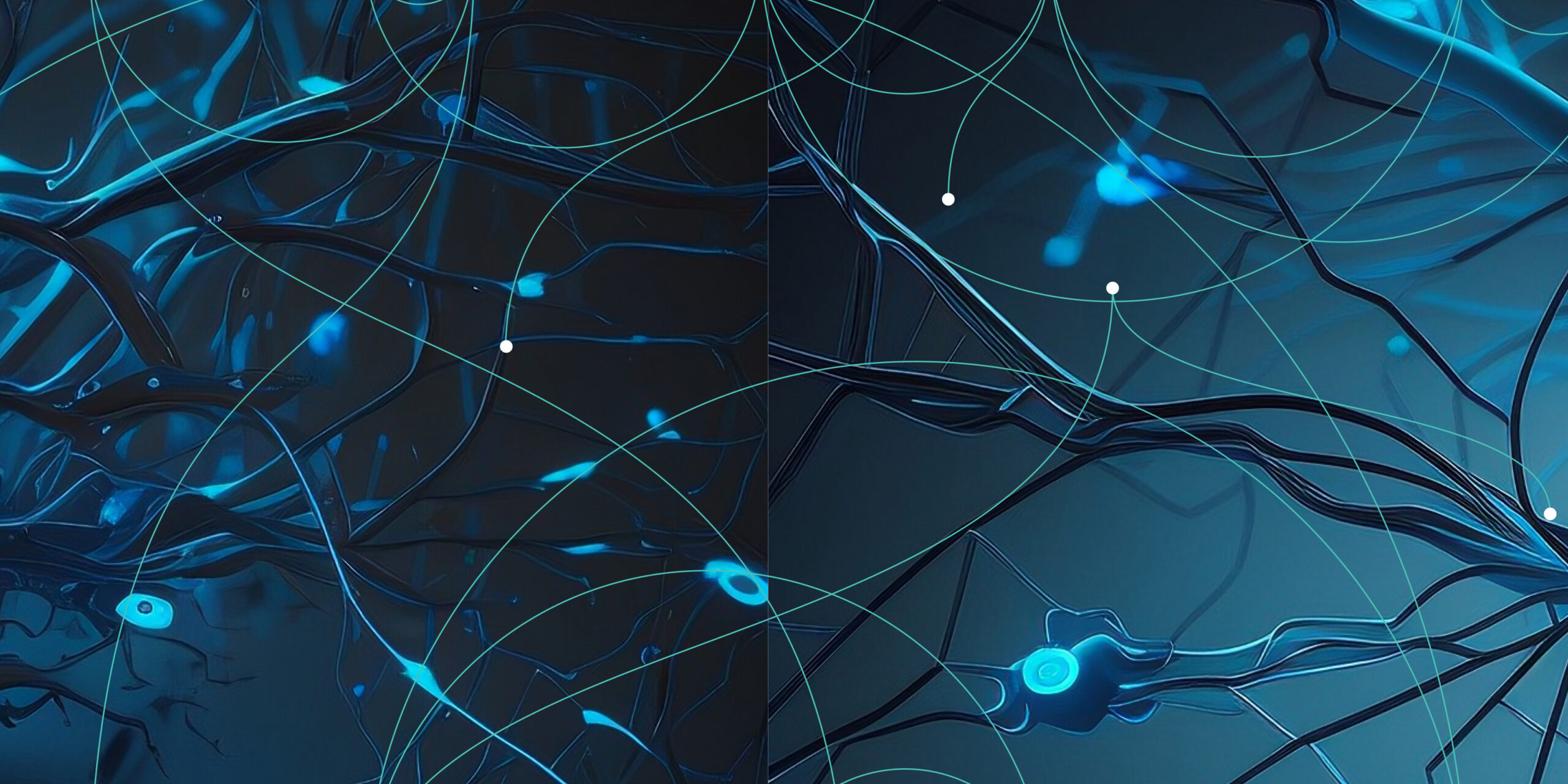
Robust and Flexible Learning
Good generalization means drawing good inferences when tested on new, previously unseen data. ARNI will create neuroscience-inspired approaches to improve learning that are not limited to the training distribution – ones that are robust to perturbations and distribution shifts, and that can transfer across tasks. New theories will be developed to rigorously analyze learning approaches.
Research Projects
Robust & flexible representations under distribution shift
Principals of flexible and robust decision making using multiple sensory modalities
Models and mechanism of abstraction
Geometric properties of disentangled neural codes
Advancing SAR target recognition through self-supervised learning: A vision for enhanced ATR systems
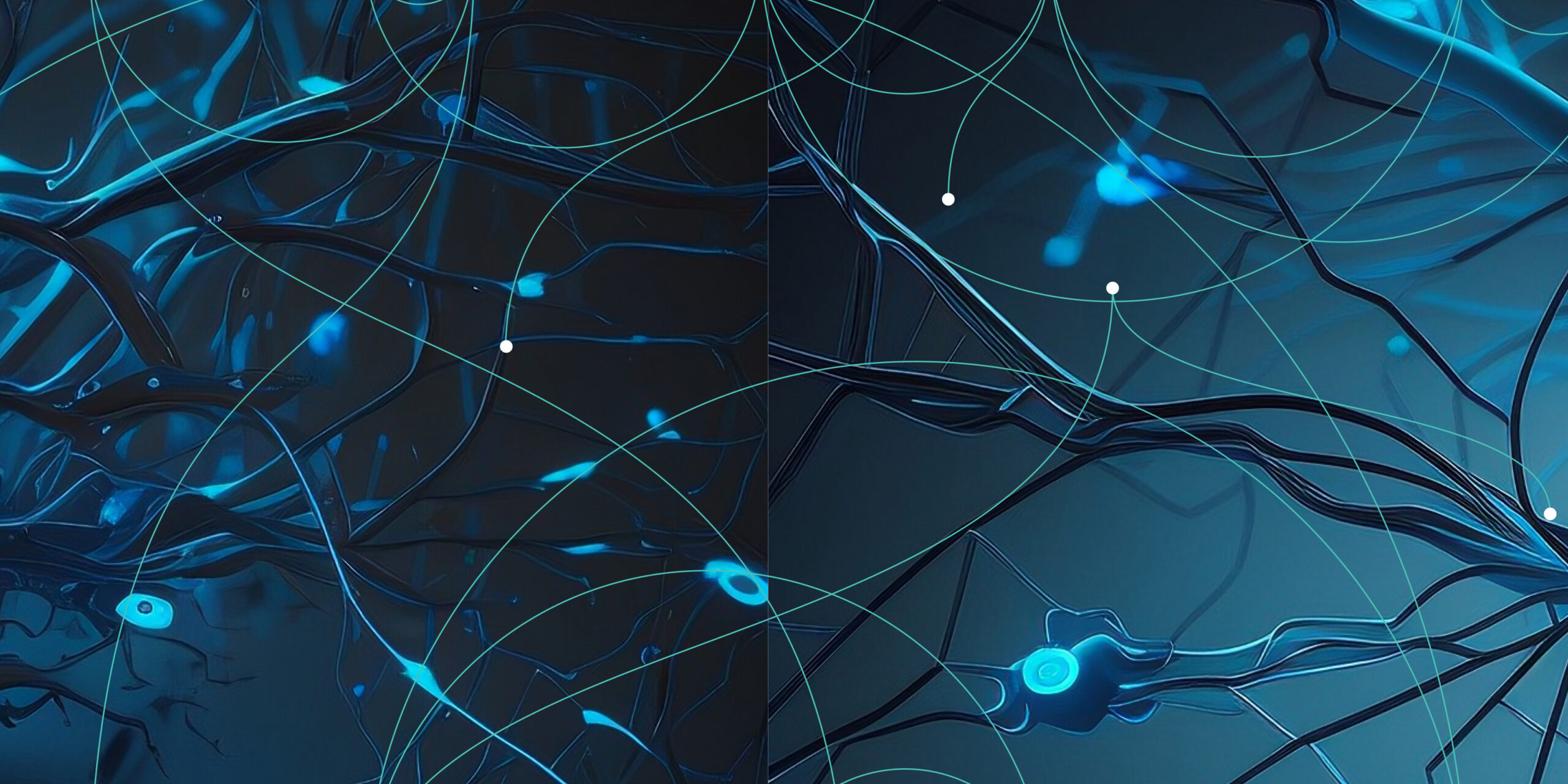
Reasoning about Causality and Uncertainty
Achieving a truly reasoning AI remains a grand challenge of AI/ML research. Despite high predictive accuracy, AI lacks causal understanding and can make overconfident, mistaken predictions. This can have disastrous consequences for real-world applications such as self-driving cars. ARNI will develop AI systems that incorporate and infer causal structures, informed by and informing new neurobiological studies.
Research Projects
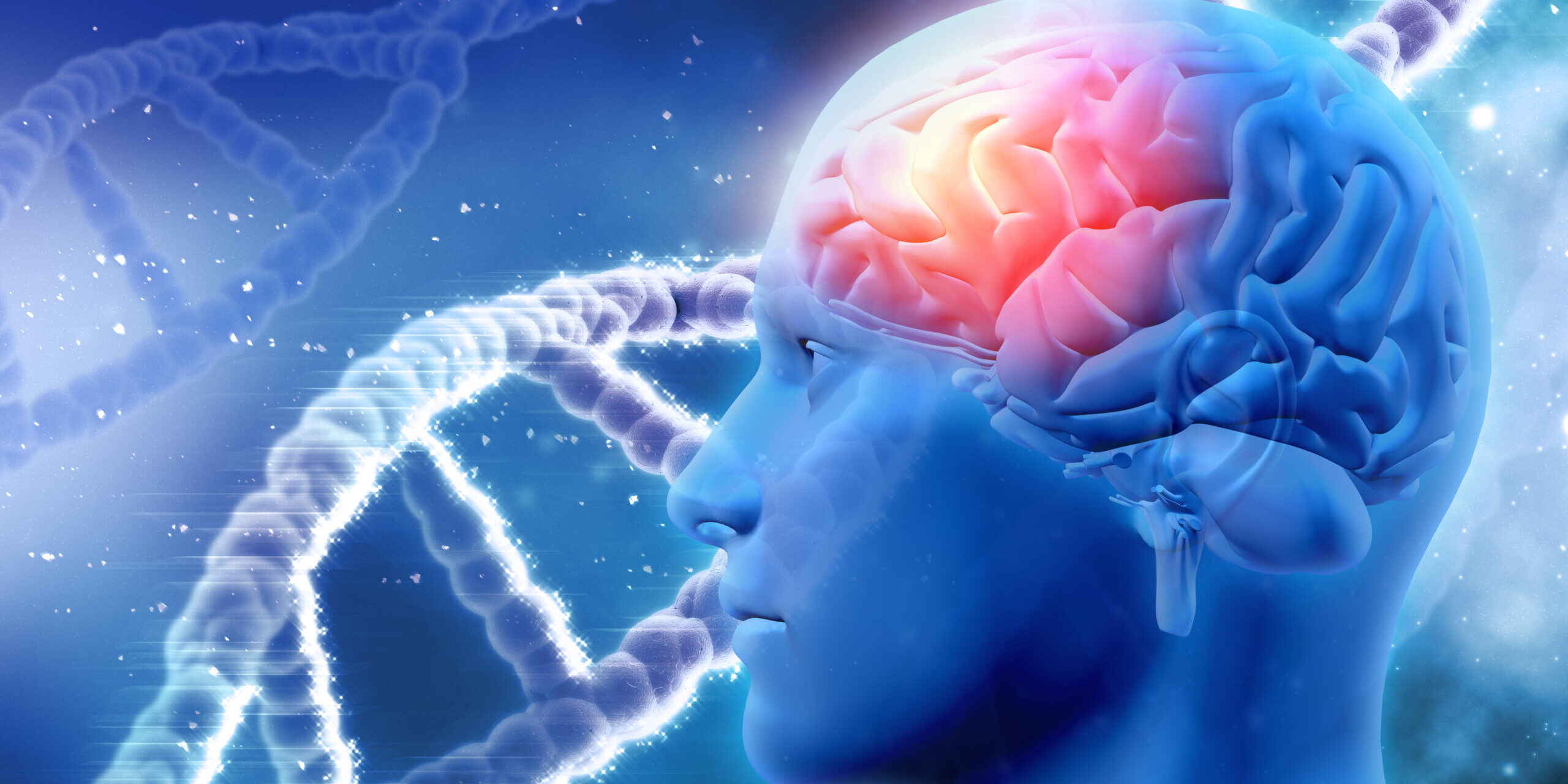
Neural Mechanisms
The brain’s machinery determines its inductive biases, which define how it generalizes. ARNI will study how biological mechanisms affect cognitive processing, generalization, and continual learning for AI, while our AI studies will produce novel hypotheses for neuroscience.
Research Projects
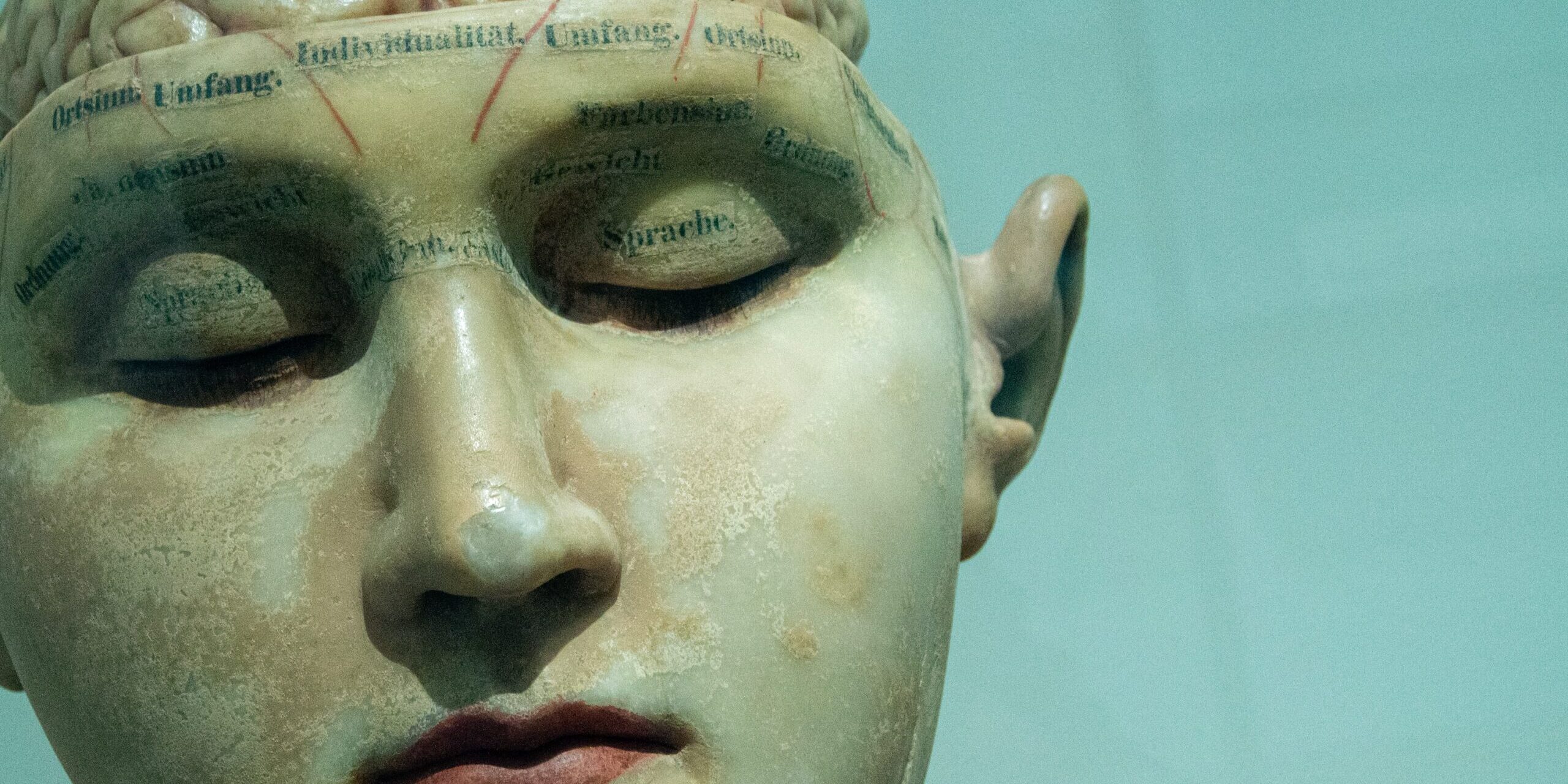
Language and Vision
The modern era of AI has been defined over the past decade by breakthrough results in two domains: vision and language. ARNI will further these to produce adaptable, self-supervised systems with continual, multimodal input which can deal with uncertainty and causality, be cognizant of cognitive processes, and informed by the state of the art in Neuroscience and Cognitive Science.
Research Projects
Understanding linguistic and visual factors that affect human trust perception of virtual agents
Understanding creative communication and making it accessible
Artificial and natural visual problem solving with resource constraints
A brainwide “universal translator” for neural dynamics at single-cell, single-spike resolution
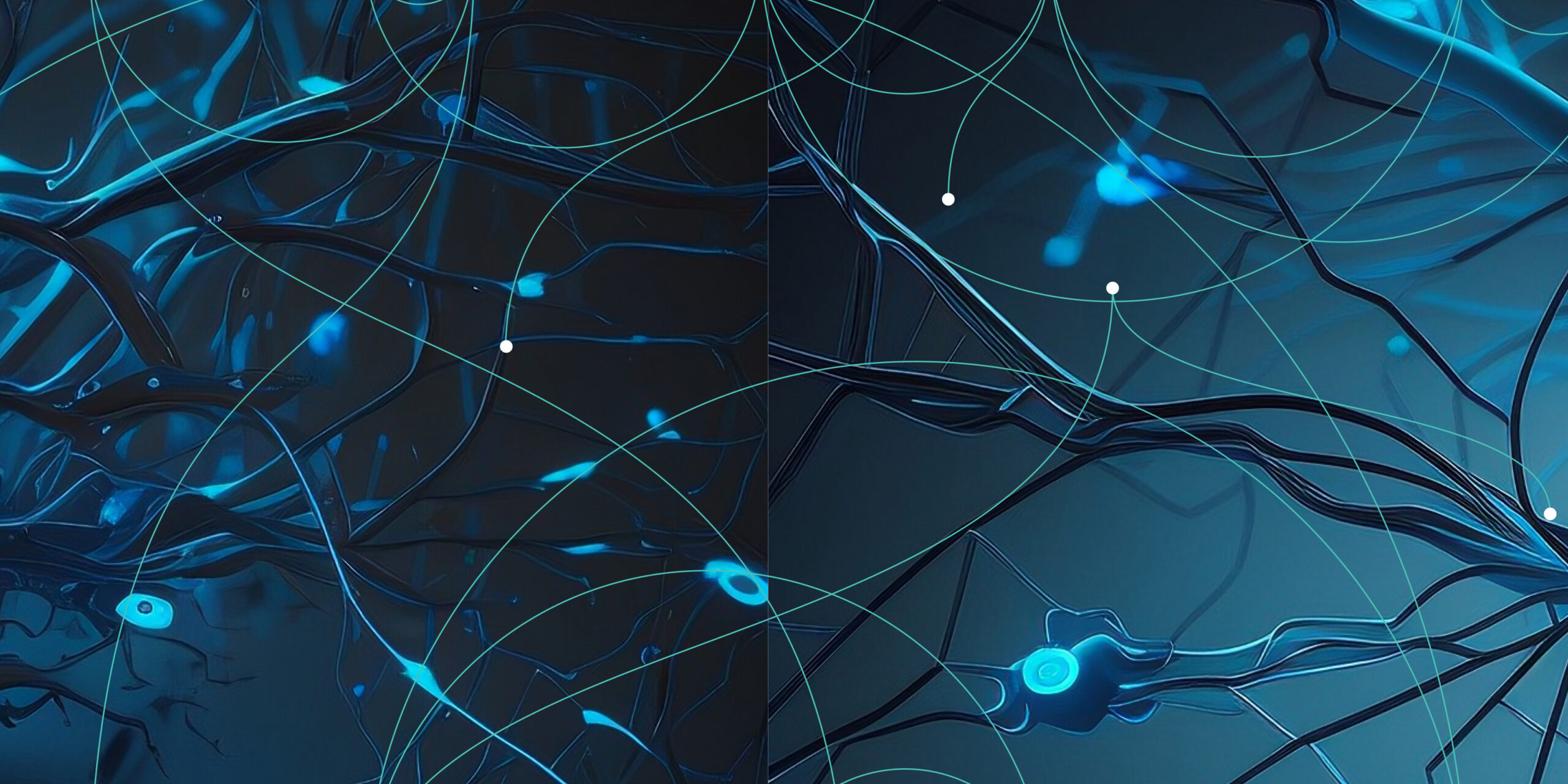
Continual Learning
Recent AI models are capable of generalizing to a remarkable range of new tasks, but often contain billions of parameters and rely on batch training with terabytes of data. In contrast, natural learning is continual, online, interactive, and adaptive to new contexts, forming a closed loop between behavior, perception, and learning. ARNI will uncover and apply insights from natural learning, which is data-efficient and leverages the rich structure of unlabeled experiential data.
Research Projects
Use-Inspired Research
In addition to foundational research, ARNI will advance the state of the art in three use-inspired research areas: societal, scientific, and industrial.
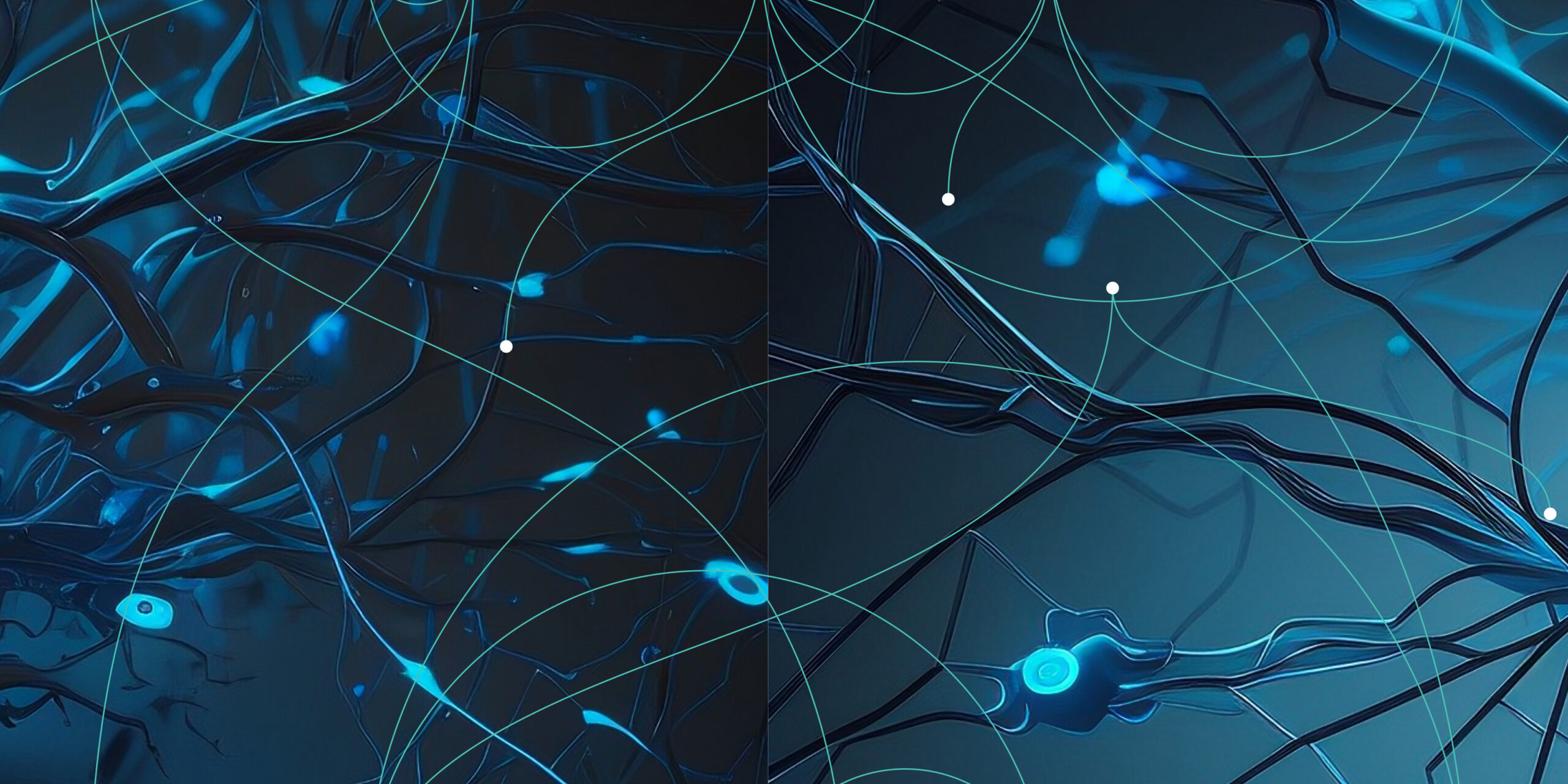
Industrial Uses
In industrial applications, a major challenge is developing machine learning algorithms to handle dynamic datasets and learn continuously in real-world environments. ARNI will create AI systems that will impact the design of tools like mobile assistants and recommender systems, and will develop use-inspired datasets to evaluate our novel learning algorithms.
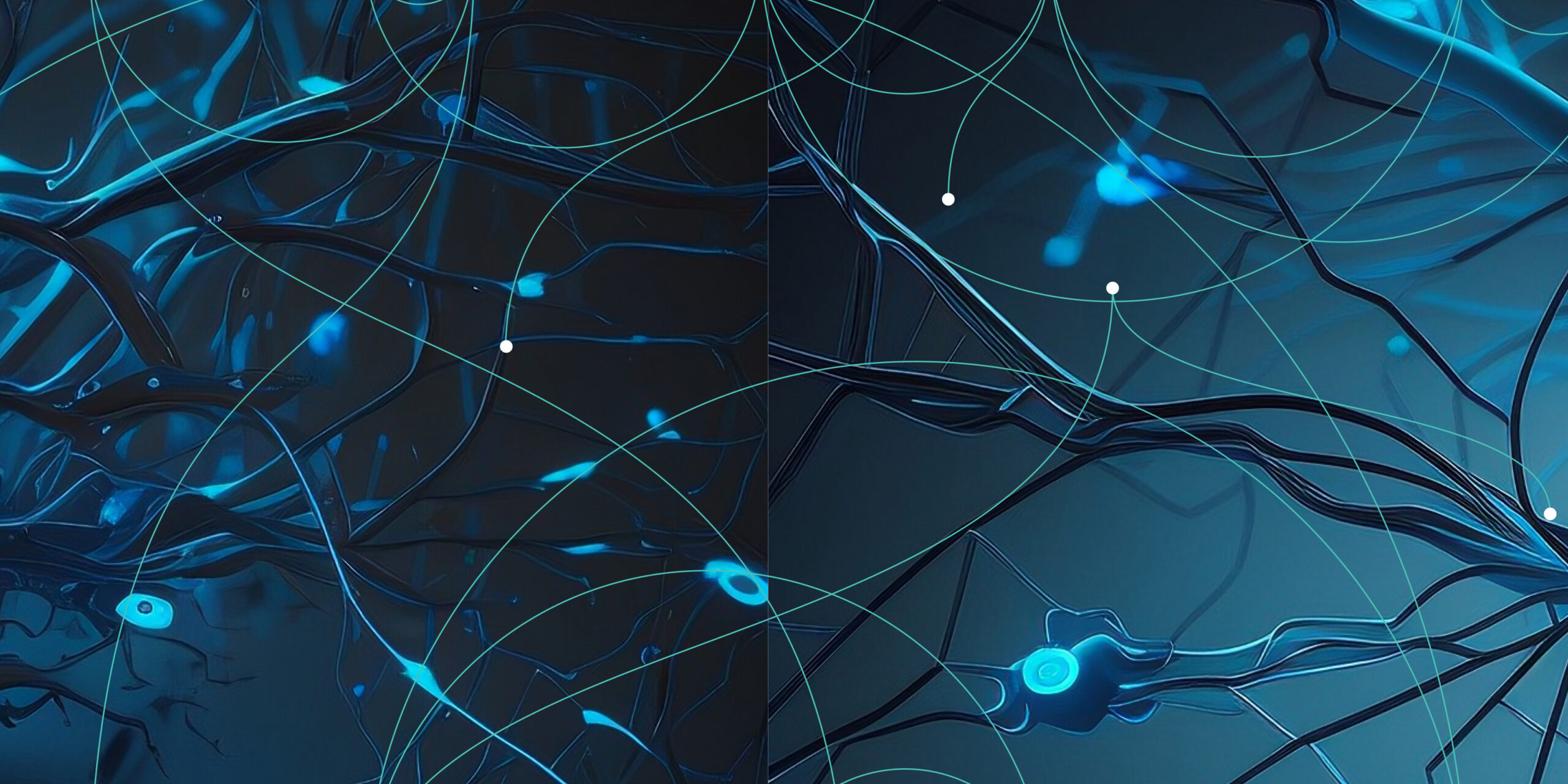
Scientific Uses
ARNI’s foundational research will help develop stronger tools for recording and decoding neural activity in the context of rich behavior. These advances in turn will accelerate developments such as brain activity decoding and architectures for computer vision.
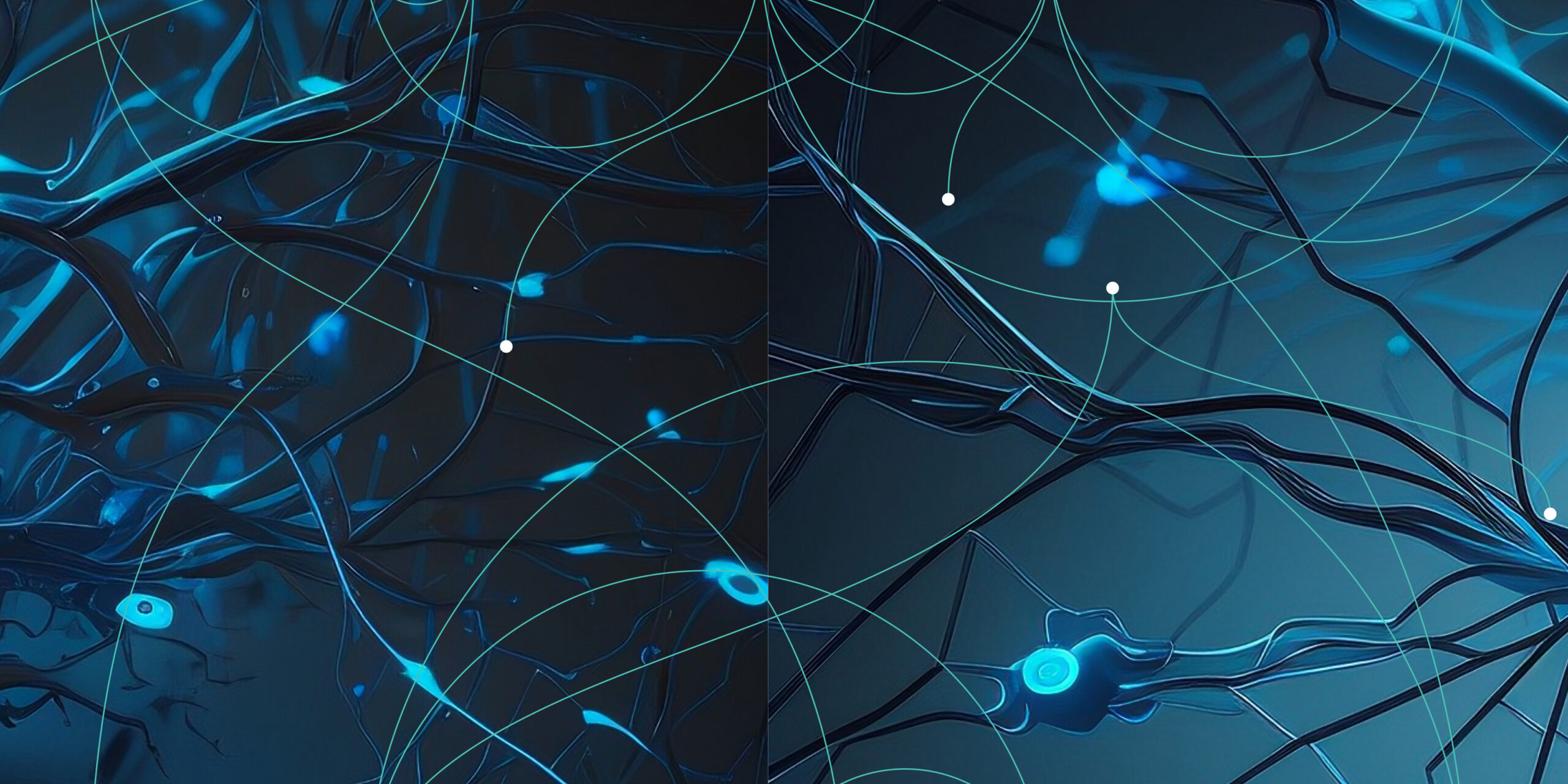
Social Uses
Algorithms that incorporate understanding of neuroscience can be used to create improved assistive technologies, and detect and deploy interventions for cognitive states in online and multimedia spaces.